
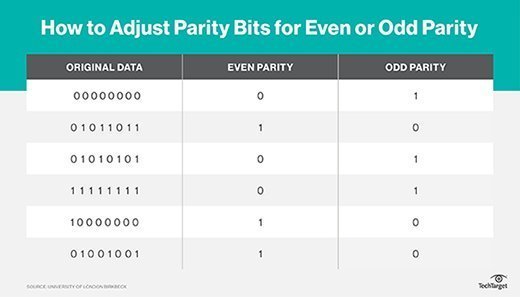
Negative predictive value (NPV) = Σ True negative / Σ Predicted condition negative Positive predictive value (PPV), Precision = Σ True positive / Σ Predicted condition positiveįalse discovery rate (FDR) = Σ False positive / Σ Predicted condition positiveįalse omission rate (FOR) = Σ False negative / Σ Predicted condition negative Prevalence = Σ Condition positive / Σ Total populationĪccuracy (ACC) = Σ True positive + Σ True negative / Σ Total population In particular, it organizes measures the population it conditions on (i.e. The table below nicely summarizes the most common evaluation metrics. Quantitative metrics for evaluation ¶įirst, we’ll review measures for evaluation of a classifier, as these are technically and conceptually the building blocks of parity measures. Recall how each of these are depend on human choice and hide the complex, dirty, processes of measurement and interpretation.ĥ.2. The decision making classifier \(C(X,A)\). The binary outcome variable denoted \(Y\), The observed characteristics of an individual (variables) be denoted by \(X\),Ī category containing a salient group (e.g. We fix notation for this context, which will be used in the remainder of the chapter: Much of this perspective follows Chapter 2 of. Such a context provides a simple, yet important, example to begin understanding these ideas. In this lecture, we will focus on allocative algorithmic decision made by a supervised, binary classifier. On the other hand, identifying such differences is a first step in surfacing possible inequities and understanding why they exist (e.g. Observational criteria, like identifying differences the distribution of salaries between men and women, don’t assert anything meaningful about the nature of the discrepancy, about why such differences exist, or whether they constitute discrimination.

We begin with observational criteria: measurement of what exists and is observable. We will now look specifically at preliminary notions of fairness applied to decision making systems powered by a supervised classifier.
